Quamba: A Post-Training Quantization Recipe for Selective State Space Models
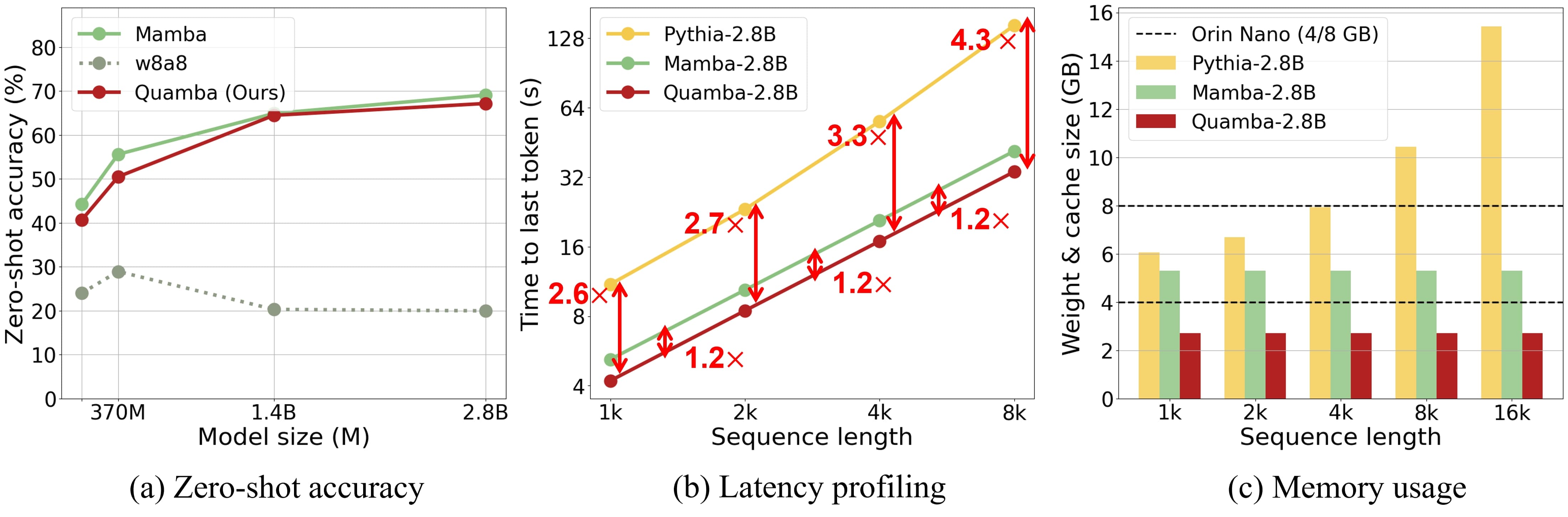
8-bit quantization (W8A8) for Mamba blocks
1.7 × speedup on Orin Nano 8G
2× memory reduction
Real-time Generation on Edge GPUs
We compared Quamba 2.8B with Mamba 2.8B on a NVIDIA Orin Nano 8G. Quamba (W8A8) is \(1.7\times\) faster than Mamba (FP16) on the Nano. The real-time generation speed is shown in the demo.
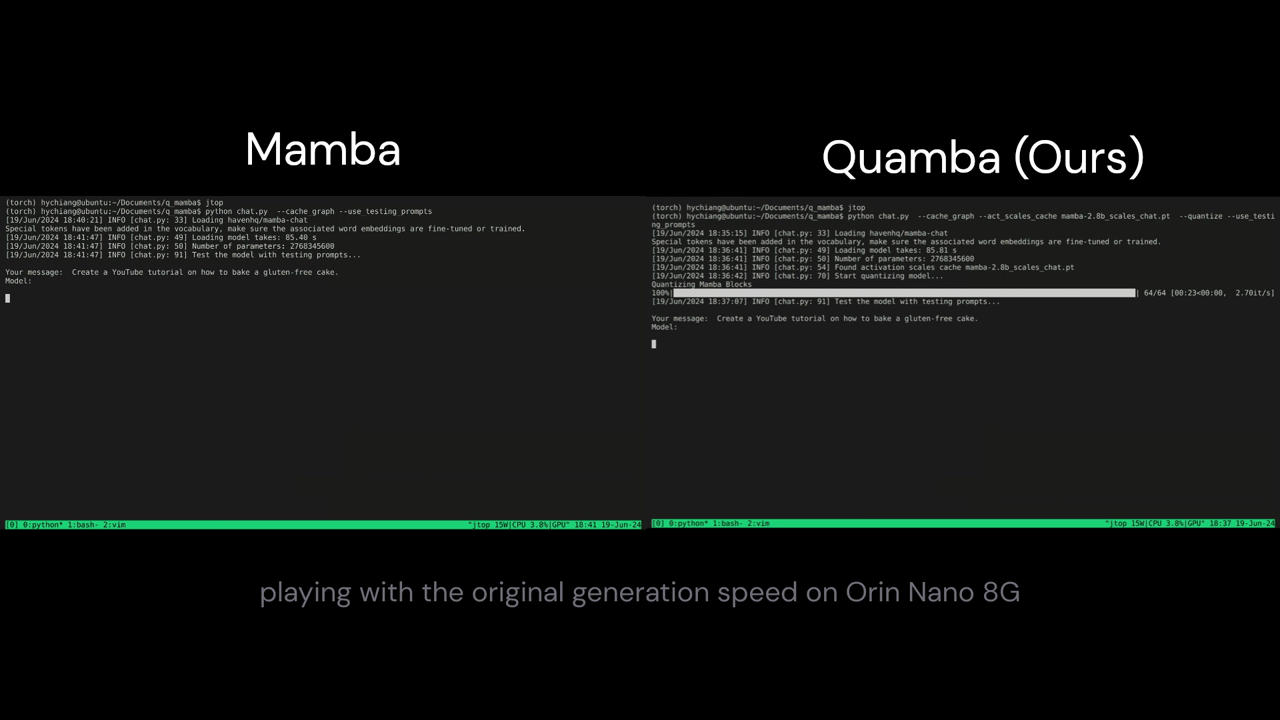
Long Input Sequences on Edge GPUs
We compared Quamba with an 8-bit transformer on a NVIDIA Orin Nano 8G. Quamba is capable of handling long input sequences (over 8k tokens) with limited memory and computational resources on edge devices.
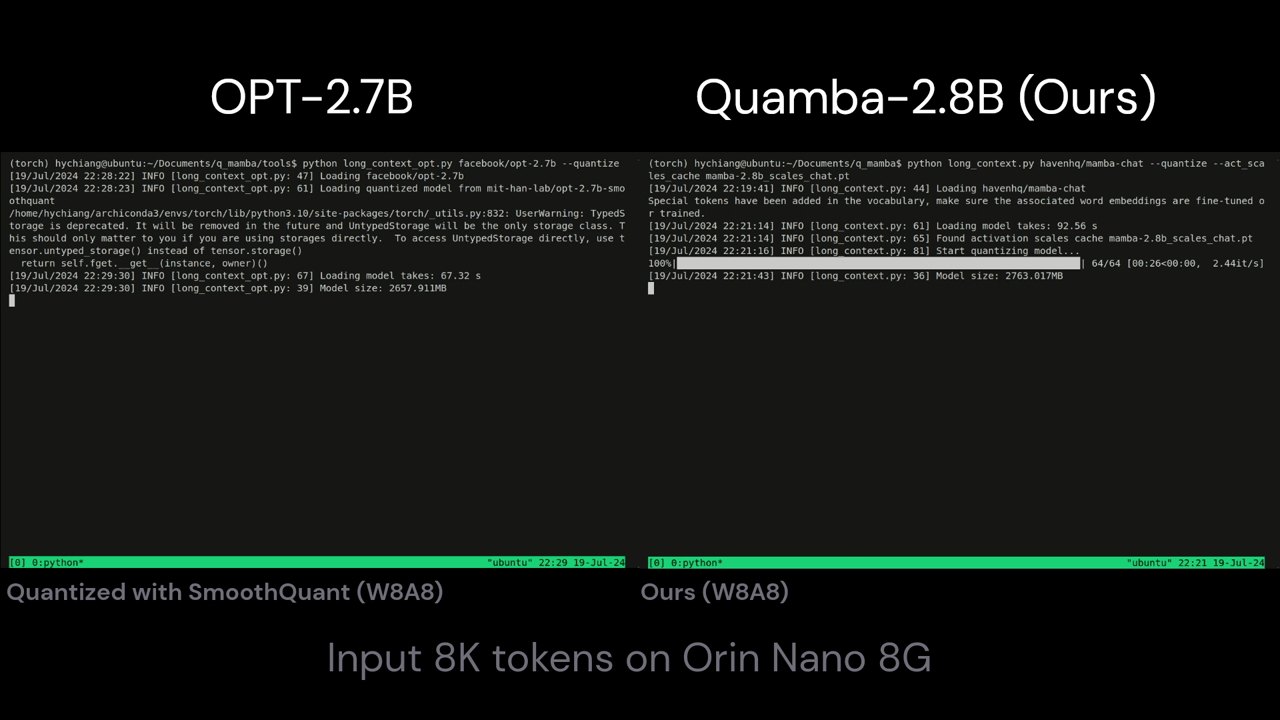
Zero-shot Accuracy
Zero-shot accuracy of quantized models on six common sense tasks. Quamba is a static per-tensor quantization method that closes the performance gap and outperforms the same-sized Transformers (Pythia) in accuracy. (Bold is the best, and underline is the second best.)
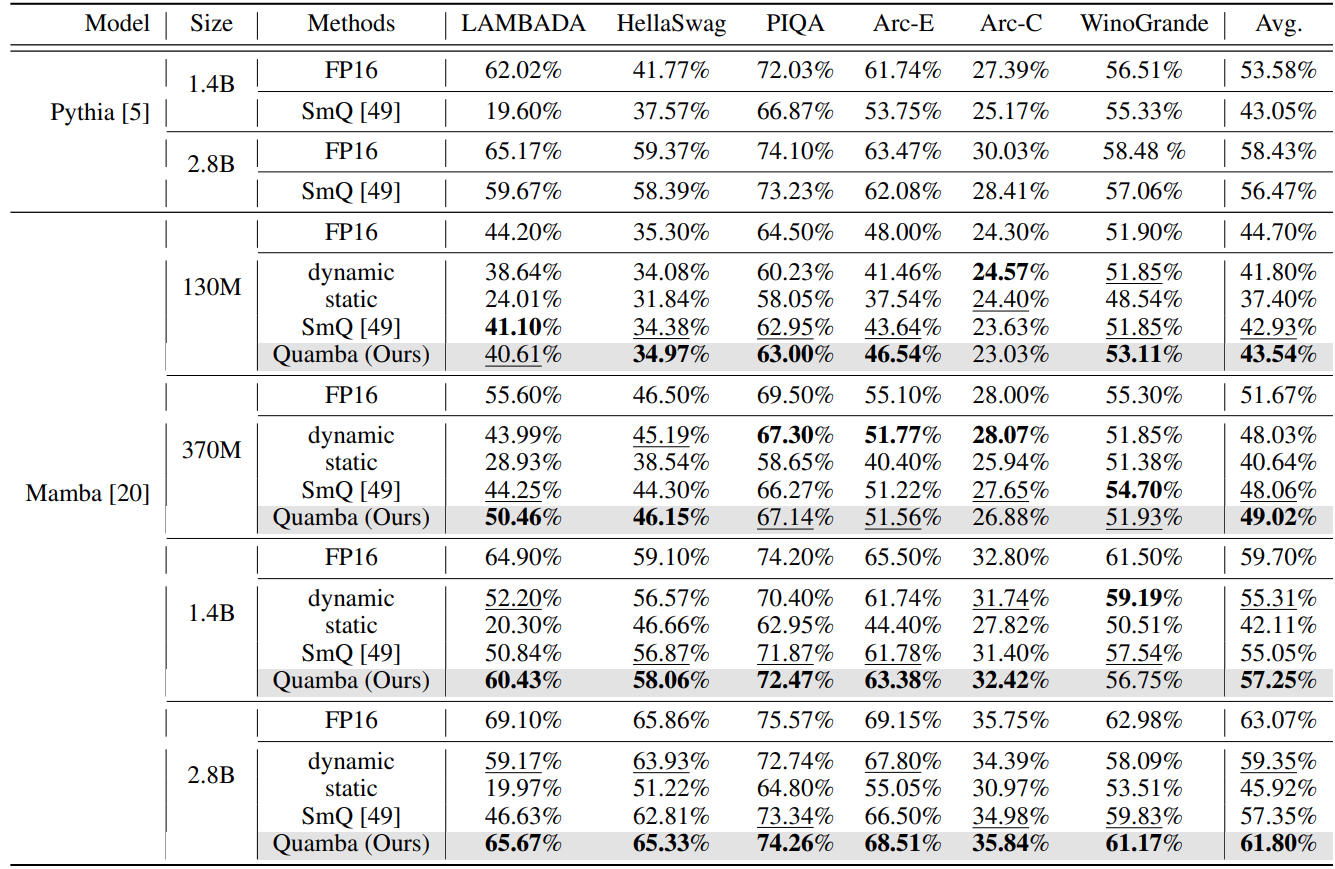
Perplexity Evaluation
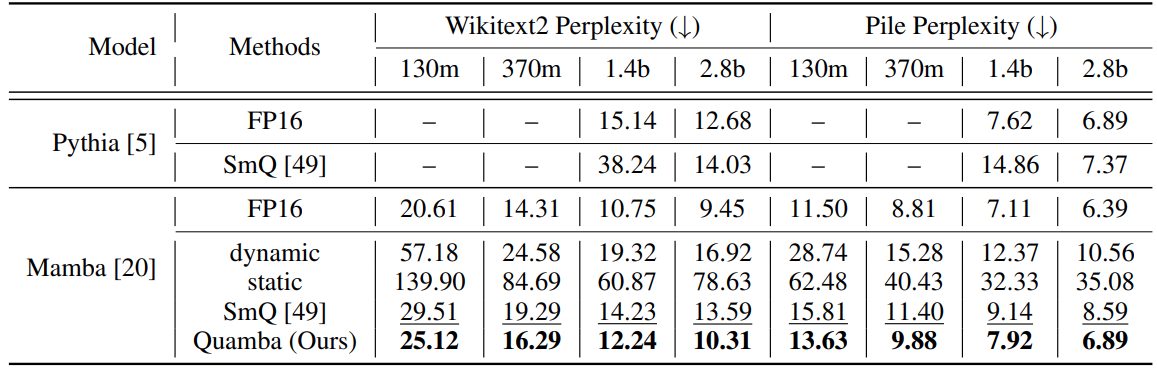
Quantizing Jamba: A Large-Scale Hybrid Mamba-Transformer LLM
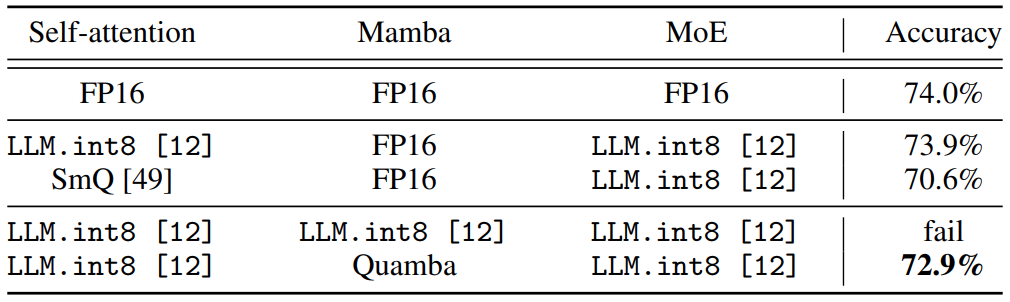
Presentation
Citation
@inproceedings{chiang2025quamba,
title = {Quamba: A Post-Training Quantization Recipe for Selective State Space Models},
author = {Chiang*, Hung-Yueh and Chang*, Chi-Chih and Frumkin, Natalia and Wu, Kai-Chiang and Marculescu, Diana},
booktitle = {International Conference on Learning Representations (ICLR)},
year = {2025},
}
Acknowledgements
This work was supported in part by the ONR Minerva program, NSF CCF Grant No. 2107085, iMAGiNE - the Intelligent Machine Engineering Consortium at UT Austin, UT Cockrell School of Engineering Doctoral Fellowships, and Taiwan’s NSTC Grant No. 111-2221-E-A49-148-MY3.