Quamba2: A Robust and Scalable Post-training Quantization Framework for Selective State Space Models
ICML 2025
Supports W4A8 / W4A16 / W4AX / W8A8 for Mamba1 and Mamba2
Up to 4× memory reduction
13 Token-per-second on Orin Nano 8G with Mamba2-8b
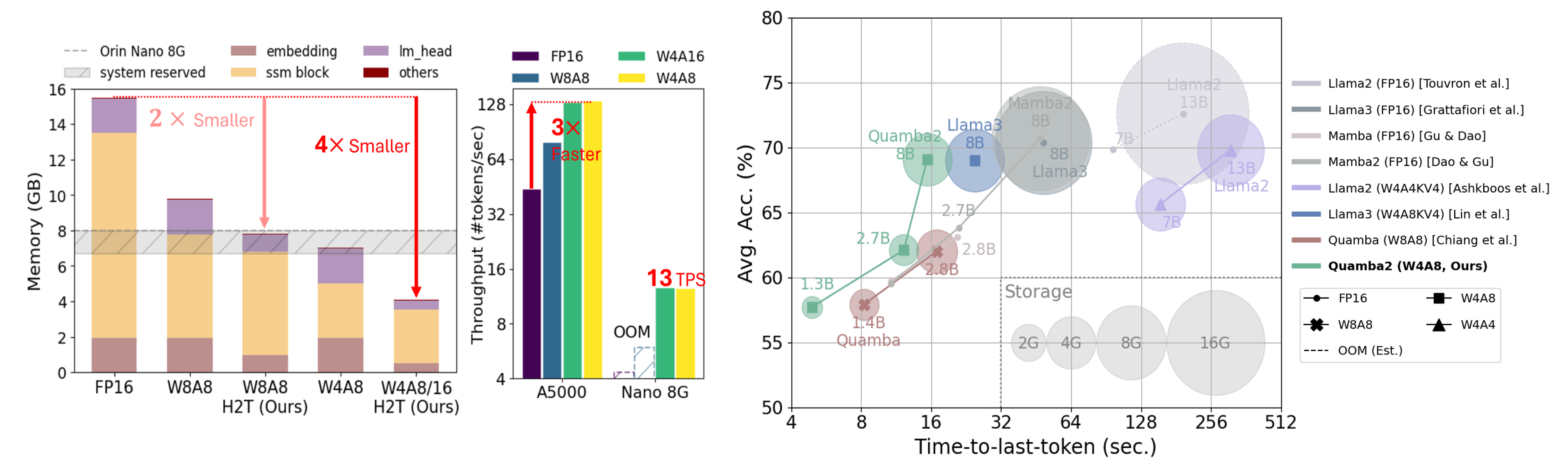
4-bit Mamba1 and Mamba2 blocks
- W4A8, W4A16, W4AX, and W8A8 for both Mamba1 and Mamba2
- Headto-toe (H2T) 4/8-bit quantization from the embedding layer, SSM blocks, to the final output layer

Storage reduction
- Achieve 4 \(\times\) memory reduction by Head-to-toe (H2T) 4-bit quantization
- Enable deploying Mamba2-8B on Nano 8G
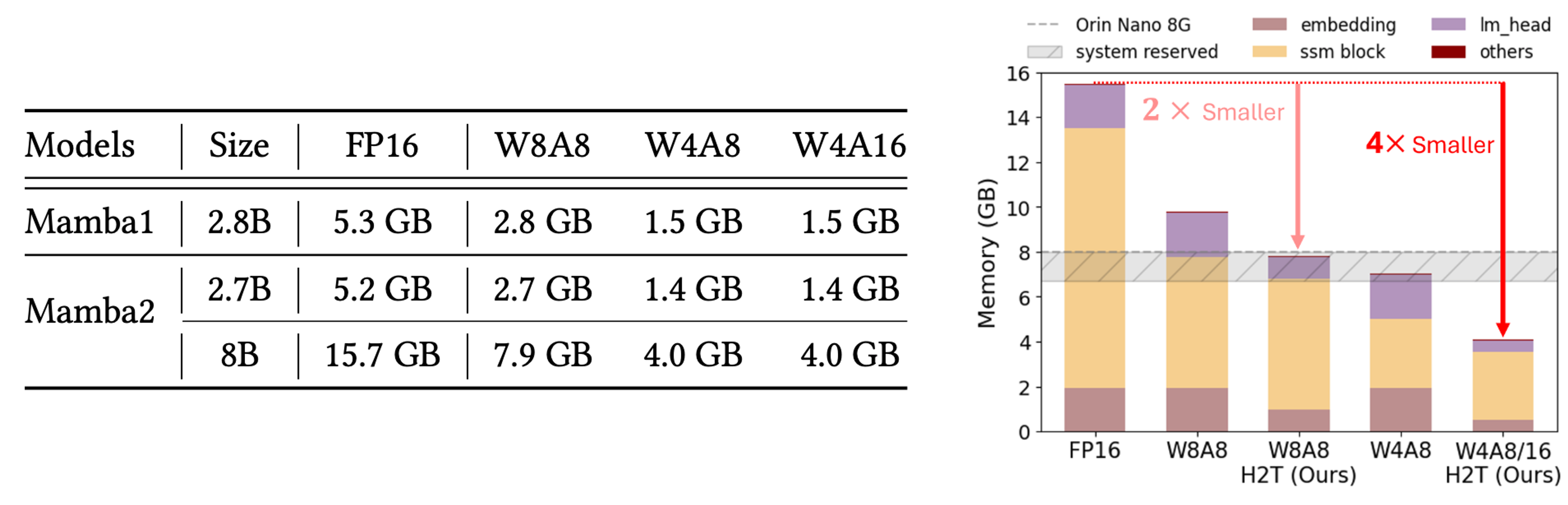
End-to-end latency speedup
- Speedup the generation by 3 \(\times\) on the A5000 GPU
- Run 13 tokens/second on Nano 8G
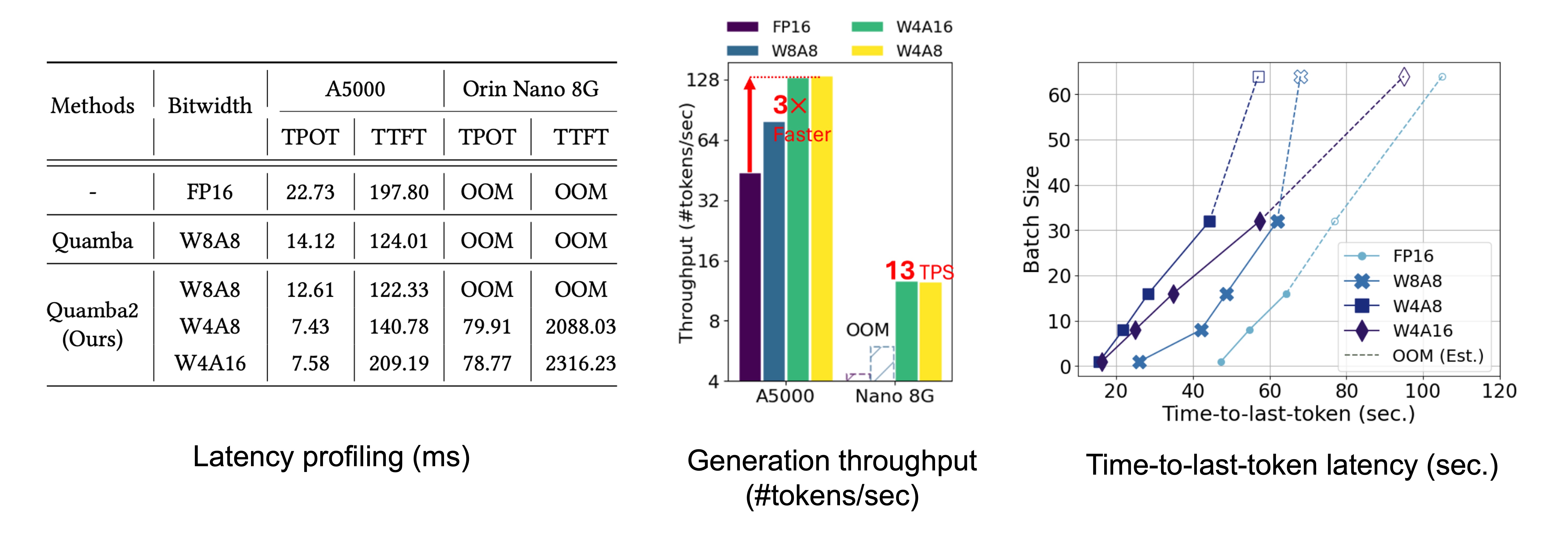
Generalization and robustness
We search W4A\(X\)-mixed (the last row in red) to improve the generalization and robustness for low bit-width SSMs. We evaluate low bit-width SSMs on the large multitask dataset MMLU.
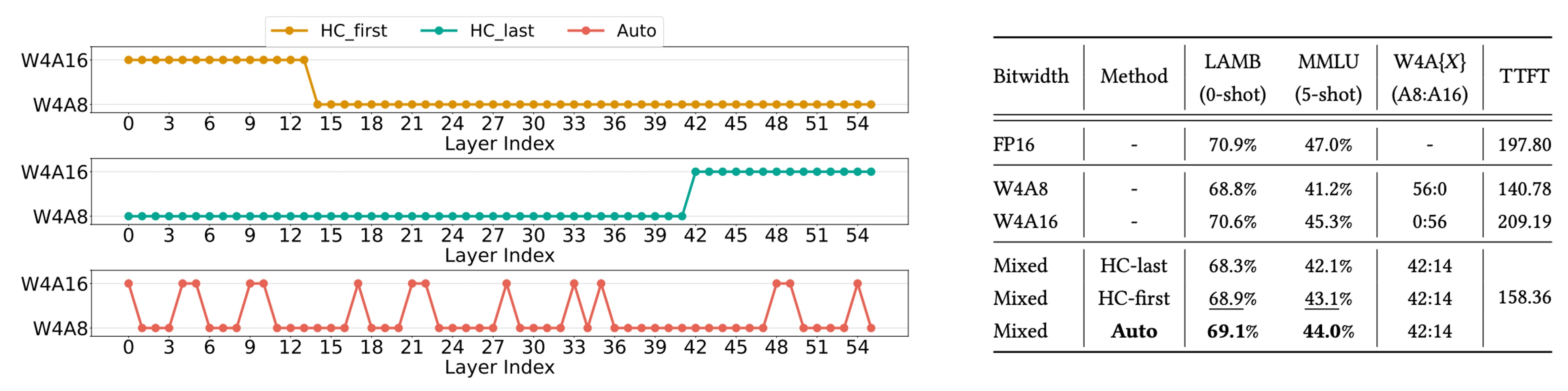
Zero-shot evaluation
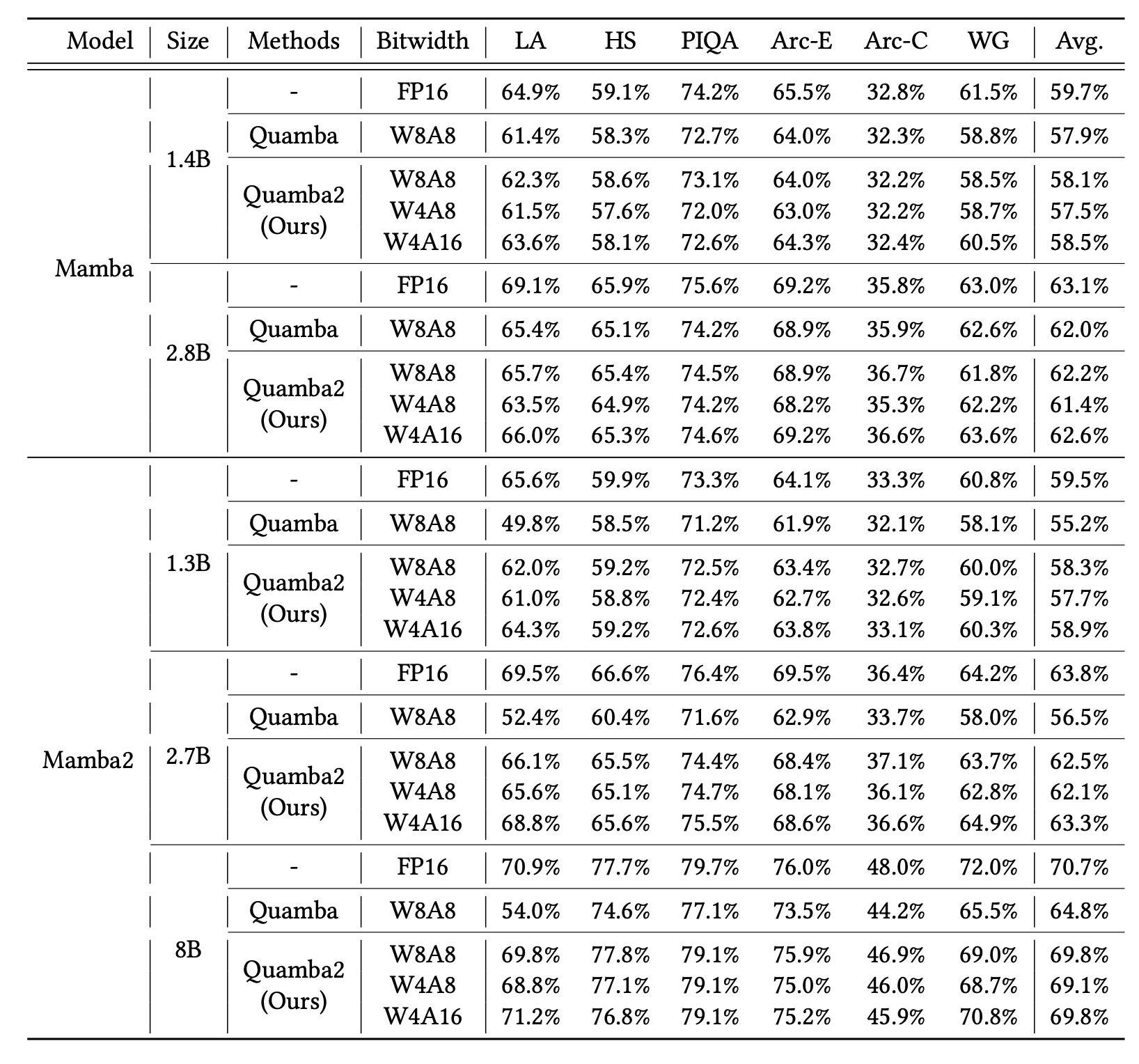
Citation
@inproceedings{chiang2025quamba2,
title={Quamba2: A Robust and Scalable Post-training Quantization Framework for Selective State Space Models},
author={Chiang, Hung-Yueh and Chang, Chi-Chih and Frumkin, Natalia and Wu, Kai-Chiang, Abdelfattah, Mohamed S. and Marculescu, Diana},
booktitle={International Conference on Machine Learning (ICML)},
year={2025}
}
Acknowledgements
This work was supported in part by the ONR Minerva program, NSF CCF Grant No. 2107085, iMAGiNE - the Intelligent Machine Engineering Consortium at UT Austin, UT Cockrell School of Engineering Doctoral Fellowships, NSF Grant No. 2339084, and Taiwan’s NSTC Grant No. 111-2221-E-A49-148-MY3.